
What is Automated Machine Learning?

The increasing rate of data inflow always leaves the analytics field around the world with a need to have a solution that can boost the efficiency of data science workflow. Every organization, regardless of its niche and size, wants to automate the repetitive work of data analysis so that data scientists can focus on other crucial aspects.
This is when automated machine learning makes its introduction.
When it comes to analyzing large volumes of data, instead of testing the skills and patience level of the data scientists, you can go for a faster and accurate way of data handling by incorporating automated machine learning in your systems. The automated process can save a lot of effort and time for data scientists. Before learning how AutoML is important for every business, let’s have a look at its brief explanation.
What is Automated Machine Learning?
Automated machine learning has completely revolutionized the way of model creation. It automates the machine learning process to solve real-world business problems with minimum manual help in a less time-consuming manner.
The solution tunes algorithms and turns the knobs in the right direction to explore which model works best for a given situation. It learns from its own data and results in the creation of effective models at a faster speed, improving the overall workflow of data science.
Benefits of AutoML:
- Keep the minds of data scientists at peace by maintaining routine tasks.
- Make computers learn how to optimize the outcome and how to perform routine operations efficiently.
- Help computers learn how to best perform each given task.
Some of the best AutoML tools are TPOT, Spearmint, Hyperopt, Auto-sklearn, and Auto-Weka.
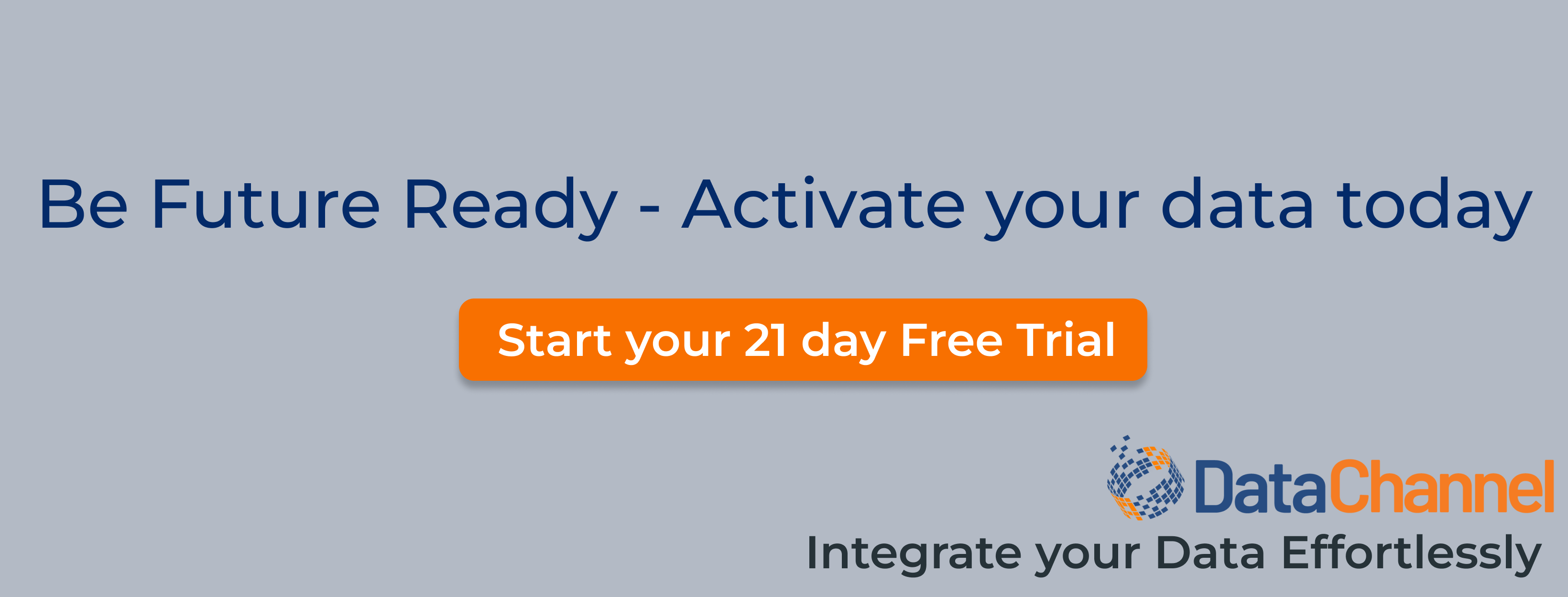
Importance of Automated Machine Learning
Manual construction of a machine learning model requires the precise following of multiple steps backed with good computer science skills, excellent domain knowledge, and mathematical expertise, which are hard to find in a single data scientist. Additionally, manual model creation involves a high degree of risk for errors & bias. This thing can hamper the overall accuracy level of the model, along with the quality of insights the model will provide. To bring accuracy to the model creation process, AutoML plays a significant role. With automated machine learning and expertise of data scientists, you can make your systems develop capabilities without spending excess time and money. The solution will assist you in improving ROI in the data science initiative by providing valuable insights at a faster pace.
AutoML can serve its utilization in different industrial segments, such as banking, finance, healthcare, marketing, sales, manufacturing, sports, and many others. It automates the modeling tasks required to develop and deploy efficient machine learning models. With AutoML, any organization can implement a machine learning solution as seamlessly as possible and allow the data scientists to work on more crucial business problems.
How to make the best use of Automated Machine Learning?
All analytics-based enterprises make use of machine learning in processing their routine tasks. Still, there are some complex tasks, such as selecting appropriate features and model family, data pre-processing, model hyperparameters optimization, post-processing of machine learning models, and analyzing results that fall way out from the league of analysts that do not have expertise in ML.
This is where automated machine learning saves the analysts from processing the mentioned applications by creating efficient models that can achieve good accuracies at the lowest computational cost possible.
Summing up
With Automated Machine Learning, data scientists and analysts can lower their dependency on intuition by trying out an algorithm, scoring, testing, and refining other models. The solution can effectively automate the machine learning process and add accuracy and effectiveness in the workflow of data science applications in every organization, irrespective of its size.
The future of data science will be all about AutoML, where the model creation and deployment process will become a piece of cake for analytics-based organizations. Once the data is set in a precise format, the automated machine learning system will be able to create and optimize the machine learning pipeline at a rapid speed, unlike humans who take multiple hours to do the same. It will also be able to process the data cleaning process, taking this responsibility as well from the shoulders of data scientists.