
Vector Databases: A Primer

Since the launch of ChatGPT by OpenAI, there has been an explosive surge in organizations eager to dive into Artificial Intelligence (AI). Beyond the simplistic chatbots, the rise of Generative AI and the development of Small Language Models (SLMs) signal that 2024 is a pivotal year for AI innovation. This year promises to be a landmark period, marked by groundbreaking advancements that will redefine the boundaries of what AI can achieve.
Thanks to the ongoing excitement around artificial intelligence, many people are becoming familiar with its key terms. One such term is vector databases, which are intricately connected to the world of Large Language Models (LLMs), Machine Learning (ML), and AI, including ChatGPT. These databases play a crucial role in managing and optimizing the vast amounts of data that power these advanced systems. As AI continues to evolve, understanding the function and importance of vector databases becomes increasingly essential. They are the silent engines driving the efficiency and accuracy of AI applications, making our interactions with technology more seamless and intelligent. Whether you’re a tech enthusiast or just curious, diving into the world of vector databases unveils the sophisticated machinery behind the magic of AI.
In our in-depth series on Vector Databases, we'll explore everything you need to know about these cutting-edge systems. Discover the 'What, Why, and How' behind vector databases, understand their distinctions from traditional databases. We'll also delve into their diverse use cases, showcasing their practical applications, and highlight the best vector databases available in the market. Whether you're a seasoned data professional or just curious about the future of database technology, this series promises to be both informative and engaging.
Let’s dive right in!

What are Vector Databases?
A vector database is a database that stores information as vectors, also known as vector embeddings. Vector embeddings are numerical representations of data objects that capture the semantic meaning or characteristics of the data in a multi-dimensional space. Essentially, they transform complex data types, like text, images, or audio, into fixed-size vectors of numbers. This transformation enables various machine learning models to process and analyze the data more effectively.
For example:
Text embeddings represent words, sentences, or documents in a way that similar meanings are placed closer together in the vector space. Techniques like Word2Vec, GloVe, and BERT are commonly used for generating text embeddings.
Image embeddings convert images into vectors based on their visual features, allowing for tasks like image classification or similarity searches. Convolutional neural networks (CNNs) are often used for this purpose.
Audio embeddings transform audio signals into vectors that capture characteristics like tone, pitch, and rhythm, facilitating tasks such as speech recognition or music recommendation.
Vector embeddings simplify complex data, making it easier to perform tasks like clustering, searching, and comparing within large datasets.
It leverages the power of these vector embeddings to index and search across a massive dataset of unstructured data and semi-structured data, such as images, text, or sensor data. Vector databases are built to manage vector embeddings offering a complete solution for managing unstructured and semi-structured data.
A vector database organizes data through high-dimensional vectors. High-dimensional vectors contain hundreds of dimensions each corresponding to a specific feature or property of the data object it represents.
Why a Vector Database?
A vector database becomes important for businesses dealing regularly with unstructured data to power machine learning models and who need to be frequently involved in the search and retrieval of required data out of huge volumes of datasets. Let’s understand in a bit detail as to why vector databases are important and the capabilities that they offer better than the traditional ones will be dealt with later on in this blog.
AI and machine learning (ML) require vector databases for several key reasons:
Efficient Data Retrieval: AI and ML often work with large datasets containing unstructured or semi-structured data such as text, images, and audio. Vector databases enable fast and efficient retrieval of relevant data using vector embeddings, that allow for rapid similarity searches.
Handling High-Dimensional Data: Machine learning models, especially those used in deep learning, often generate high-dimensional vector embeddings. Vector databases are specifically designed to store and manage these high-dimensional vectors, ensuring efficient data processing and storage.
Scalability: AI and ML applications frequently involve scaling up to handle massive amounts of data. Vector databases are optimized for scalability, enabling them to manage and process large datasets without significant performance degradation.
Similarity Searches: Many AI applications, such as recommendation systems, natural language processing, and image recognition, rely on finding similar items within a dataset. Vector databases use techniques like nearest neighbor search to quickly find and retrieve similar vectors, enhancing the performance of these applications.
Improved Performance: By leveraging vector embeddings, vector databases can perform complex queries and analysis tasks more efficiently than traditional databases. This improved performance is crucial for real-time AI applications where speed and accuracy are essential.
Versatility: Vector databases can handle various types of data, making them versatile tools for different AI and ML tasks. Whether it's text, images, or audio, vector databases provide a unified solution for managing and querying diverse datasets.
Vector databases can be used to create unique experiences for users on mobile devices as well. For example, similar photographs on a mobile device can be grouped together into a single folder based on similarity features (such as face detection, location and time stamp of the photos clicked).
Furthermore, teams can also use different types of Ml models available to support different types of metadata extraction. With enhanced metadata extraction users can go for hybrid search results based on embeddings created for different images, documents, other forms of written content, etc. These vector embeddings can then be used to deliver more distilled & better matched results.
Vector databases enable hybrid searches that can be used to deliver nuance results using generative models. One such example is Midjourney that can be used to generate high definition images out of simple, defined descriptions or Chatgpt that cna easily generate long lines of custom code for users.
How does a Vector Database Work?
A vector database relies on a process called Approximate Nearest Neighbour (ANN) search, which identifies the vector embedding closest to the search query. While this method processes results quickly, it is less accurate than a KNN (known nearest neighbor) search. Vector databases are ideal for large datasets, where ANN search excels at processing and retrieving information more efficiently than traditional databases.
To deliver the desired output, vector databases use techniques like hashing, quantization, or graph-based search. These methods will be discussed in more detail later in this series. Vector databases depend on vector embeddings to generate answers to questions or prompts. As previously mentioned, a vector embedding is a mathematical representation of data, whether it's text, images, videos, or other unstructured data types.

The process begins with creating vector embeddings, as shown in the above image. After the embeddings are created, the next step is 'vectorization,' where vectors generated using an appropriate embedding model are placed closer together in a high-dimensional space based on context and semantics. Vectors that are closer together are more similar and will be grouped next to each other when generating an output.
The following step is 'indexing,' where the vector database indexes vectors using algorithms like PQ, LSH, or HNSW. The specifics of these algorithms and the mechanics of vector indexing will be explored in upcoming blogs.
Finally, in the 'querying' stage, a user’s query is first converted into a set of vectors using an embedding model. These queried vectors are then compared with the indexed vectors (already available) based on nearest neighbor search, and the desired output is generated, whether in the form of text, audio, or other media.
How do Vector Databases differ from Traditional Databases?
Traditional databases operate using KNN (Known Nearest Neighbour) approach and store information in a two-dimensional tabular format. When queried, they return an exact match for the query. However, traditional databases are not well-suited for handling larger datasets or for applications involving ML/AI or generative models.
This is where vector databases excel, as they are designed to efficiently query large volumes of data using approximate nearest neighbor search. Vector databases support high-dimensional search, build custom indexes, and offer flexibility and scalability.
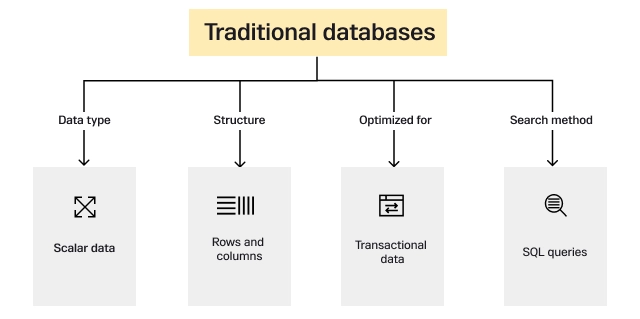
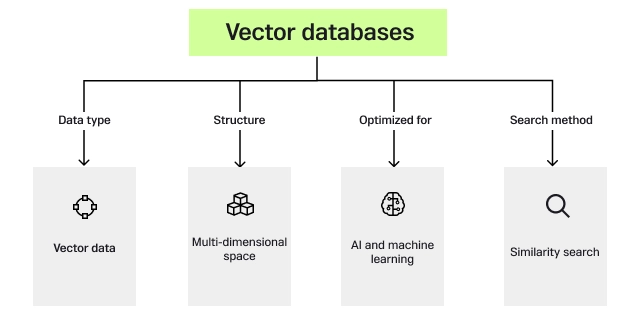
Unlike traditional databases, vector databases are inherently suited for similarity searches required in NLP (Natural Language Processing), reinforced and supervised learning, etc. The image above very well highlights the differences between traditional and vector databases.
That's it for the first part of our series. We hope you now have a better understanding of vector databases, why they are needed, how they differ from traditional databases, and a brief overview of their functionality. In the upcoming blogs, we'll delve deeper into their workings, use cases, and their essential role in modern tech stacks. Stay tuned for more. Meanwhile, if you're ready to start your data journey with DataChannel book a call with us to learn more.